AI: Benefits That Extend Beyond the Reporting Room?
Of the growing number of artificial intelligence (AI) applications to the field of healthcare, the domain of medical imaging has seen the most development. Seen in the volume of published literature1, examples of real-world deployments2, and invigoration of the diagnostic imaging workforce, radiology is perhaps best positioned of all the medical specialities to take immediate advantage of the great promise of AI. Encouragingly, there is a mounting body of evidence to suggest that deep learning models in particular can assist radiologists with disease detection3, reporting times4, and workflow efficiency. However much less work has been done examining the benefits of AI enabled medical imaging tools beyond the radiologist reporting room.
As medical imaging forms a cornerstone of clinical medicine, informing diagnostic and management decisions within all areas of the healthcare system, AI enabled gains to the efficiency and efficacy of these activities have the potential to be impressively widespread. Of particular relevance are under resourced care delivery settings where clinicians must make diagnostic and management decisions with the benefit of medical imaging, but without immediate availability of specialist radiologist opinions.
In addition to assisting with image interpretation tasks, AI generated data on disease incidence and prevalence can be leveraged through integration with existing information technology infrastructure and electronic medical record systems. In doing so, it opens further applications within population health, safety and quality assurance, research and beyond. An understanding of these wider applications of medical imaging AI systems, and the challenges associated with their adoption and validation, should be of particular interest to prudent administrators, inquisitive researchers, and hopeful vendors alike.
CLINICAL DECISION SUPPORT
While the immediate communication of expert level image interpretation between radiologist and clinician remains the ideal, system wide challenges both within and external to the radiology department often make this impossible. Frequently observed in emergency departments, regional care facilities, and after-hours settings across the globe, clinicians hampered by time constraints and increasing workloads must make clinical decisions prior to the availability of specialist radiologist reporting.
This is particularly concerning given rates of disagreement between radiologists and emergency physicians have been observed to be between 15% and 60%5 in some cases. In such settings, the timely delivery of accurate information to assist non-radiologist clinicians with image interpretation and subsequent clinical decision-making could be of value.
AI may have a role to play in bridging this time and information gap. As they apply to the point of care setting, the prediction outputs of currently available medical imaging AI tools are threefold – the presence of findings, the location of these findings, and a numerical confidence or likelihood in these predictions. An example of these features in the context of chest X-ray interpretation can be seen in the publicly available Annalise CXR demo.
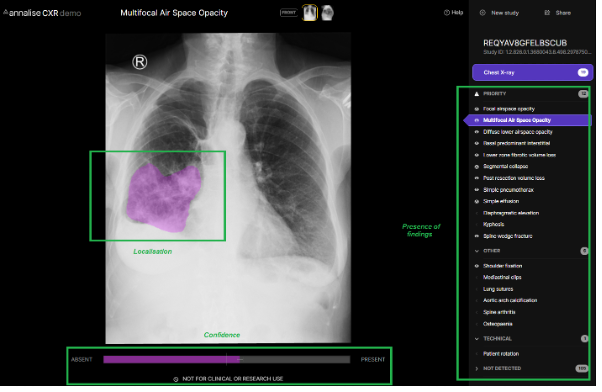
As the average processing time to generate these predictions is within seconds, the rapid availability of this information during the patient encounter would have many advantages for the treating clinician. Perhaps most obviously it could facilitate a more accurate diagnosis by affirming or querying a treating clinician’s initial interpretation – acting as a ‘second pair of eyes,’ which in many cases are able to detect radiological features at a level approaching or exceeding that of expert radiologists6. With the benefit of a clinical picture unavailable to either radiologist or AI, the treating clinician can combine highly accurate radiological interpretation with clinical acumen to arrive at better informed diagnostic and management decisions. It may also facilitate faster reading times, either by clarifying an otherwise difficult or equivocal study, or by reducing the need to seek busy senior colleagues for second opinions in the case of simpler images being interpreted by junior clinicians.
Conceivably, faster and more accurate imaging interpretation at the point of care could have positive downstream consequences on treatment times, misdiagnosis rates, patient flow, and the overall disposition process. The degree of these changes and whether they translate to cost savings and patient outcomes will of course be dependent on the modality, the patient, and the disease in question, necessitating further research into this area.
PATIENT SAFETY AND QUALITY ASSURANCE
Further to real-time user interfaces that allow clinicians to interact directly with AI generated predictions, integration of this data into existing health information systems may unlock even greater functionality for patient safety and quality of care.
Detection of findings that warrant urgent review and management, such as a misplaced nasogastric tube, massive intracranial haemorrhage, or saddle embolus can be brought to the attention of treating clinicians by means of eMR or smartphone push notifications. When seconds and minutes matter, a strategic interruption to alert a busy clinician working in activity rich, high pressure environments could mean the difference between an adverse event and avoiding significant mortality or morbidity.
In clinical settings where emergent and acute diseases are of primary concern, clinicians can suffer from a bias toward detecting findings on imaging that relate to such pathology, at the expense of non-urgent but nonetheless important features that may require further workup – so called ‘incidentalomas’ of pulmonary, adrenal, thyroid, renal, pituitary, or ovarian origin. Similarly, subtle fractures, infections, and wayward air or fluid are easily missed on suboptimal images of uncooperative patients that give inadequate histories to the junior doctor managing multiple cases overnight. While many Emergency Medicine quality standards7 recommend regular auditing of medical imaging results to ensure important findings are not missed during the patient encounter, this retrospective results-checking process performed by specialist staff is expensive and time consuming. Manual follow up of discharged patients is rarely as effective as real time discussion at the time of the initial consultation, and follow-up may not be possible – itinerant, remote and rural and other, already disadvantaged, populations are like to be over-represented here. Missed diagnoses can have significant consequences for patients and clinicians – medicolegal concerns aside, delayed treatment and poor outcomes are of justifiable concern.
The detection of these subtle and non-emergent findings during the patient encounter, brought to the attention of treating clinicians by astute and indefatigable AI tools, permits the timely communication of these findings and their significance to the patient and an opportunity to reduce treatment delays, workforce costs, and patient morbidity that would come from otherwise missed or delayed findings.
POPULATION HEALTH AND SCREENING
The application of AI algorithms to large medical imaging databases, contained for example within hospital picture communication and archiving systems (PACS) or large-scale vendor neutral archives, may assist with much needed identification of chronic disease cohorts at the population level. Chronic obstructive pulmonary disease, cardiovascular disease, occupational lung disease, osteoporosis, and malignancy are all conditions with features detectable on imaging modalities that are of particular importance to public health agencies due to their significant and largely preventable burden of disease. Reports of worsening backlogs in unreported scans from around the world8, exacerbated by COVID 19 and disproportionally affecting low resource environments, is also likely contributing to under diagnosis and monitoring of chronic disease and presents another opportunity for AI to contribute to better health at the population level.
Identifying chronic disease sufferers through AI detected imaging characteristics could generate valuable data on the incidence and prevalence of disease, that as a first step, would enable better health services planning9. The quality of these statistics would be significantly improved by enriching AI outputs with existing patient eMR data from hospital or primary care settings. The creation of geographical disease registries identifying at risk or affected populations at the community, provincial and national levels could then facilitate coordinated population health level interventions. Targeted initiatives such as at-risk population screening, automated enrolment into chronic disease care programs, or the delivery of educational material for primary and secondary prevention would better use existing care pathways and public health initiatives. There is even potential to contribute to improvements in environmental factors for health, for example by guiding monitoring and reporting of air quality based on the geographic distribution of patients with signs of chronic lung disease.
CHALLENGES
The translation of AI systems into routine clinical practice is a challenging process without precedent. Despite a general consensus on the potential for medical imaging AI to positively impact healthcare, examples of successful deployment into clinical practice are few10, and fewer still when considering applications beyond the reporting room.
Though an impressive number of in-vitro studies confirm expert level image interpretation by machine learning algorithms, robust peer reviewed clinical evaluation demonstrating economic or clinical benefits are lacking. Peer-reviewed randomised control trials focussing on clinical and economic outcomes will be important not only for hospital administrators looking to justify AI spending, but also to build trust within the traditionally conservative wider medical community.
Difficulties in the translation of medical imaging AI into clinical practice do not end with the need for robust evidence generation. There are many logistical difficulties faced when adopting AI tools in healthcare that arise from siloed data, existing systems integration, and security concerns. Challenges unique to machine learning such as hidden stratification, generalisability, and algorithmic bias are likely to be underestimated by teams without clinical guidance. Adherence to robust regulation and rigorous quality control need to be held to the same harsh standards as all new clinical therapies and are fundamental in achieving safe and effective AI deployment.
Finally, human barriers to adoption of AI tools are substantial even when all other obstacles have been removed, with concerns about the effect of AI on the roles and responsibilities of radiologists and other clinicians into the future being a particularly sensitive topic. The benefit of artificial intelligence tools can only come from changing the behaviour of the ‘effector arm’ – the human users. Understanding where within clinical workflows we hope to create change, and how to deliver information in the most effect way to create that change is as much part of the challenge as designing algorithms. Maintaining this focus on human-computer interaction, clinical applicability, and the improvements to the lives of patients and clinicians will be crucial in making necessary headway within the medical community and the greater lay population.
CONCLUSION
Despite the challenges of translating medical imaging AI systems into routine clinical practice, there is clear potential for benefits to be felt beyond the radiologist reporting room and in the wider healthcare system. With strains on emergency departments, low resource settings, and public health systems at an all-time high, novel technologies to help alleviate these pressures will be a welcome and necessary advancement, and the implementation and validation of such tools should be a priority for health administrators and clinicians looking forward.
From the AI vendor perspective, given the substantial time and financial investment required to develop effective medical imaging systems, finding applications beyond interpretation assistance for radiologists will allow them to maximise clinical impact and return on investment. A focus on non-radiologist clinician usability, integration with health information systems used at the point of care, and comprehensive interpretation will maximise the deployment potential in the widest variety of settings.
Ultimately, robust clinical evidence that demonstrates better patient outcomes, cost and resource savings, and improvements to the experience of delivering care will act as arbiter and enabler of AI deployment in all setting. Collaborations between vendors, health services and academic institutions should be encouraged as they are the necessary step in the generation of high quality, translational research evidence to drive the adoption of innovative technologies within healthcare now and into the future.
SOURCES
1 – Pesapane F, Codari M, Sardanelli F. (2018). Artificial intelligence in medical imaging: threat or opportunity? Radiologists again at the forefront of innovation in medicine. Eur Radiol Exp, 2(1), 35.
2- Kelly, C.J., Karthikesalingam, A., Suleyman, M. et al. (2019). Key challenges for delivering clinical impact with artificial intelligence. BMC Med, 17, 195.
3 – Conant, E. F., Toledano, A. Y., Periaswamy, S., Fotin, S. V., Go, J., Boatsman, J. E., & Hoffmeister, J. W. (2019). Improving Accuracy and Efficiency with Concurrent Use of Artificial Intelligence for Digital Breast Tomosynthesis. Radiology. Artificial intelligence, 1(4)
4 – Kim JH, Kim JY, Kim GH, et al. (2020) Clinical Validation of a Deep Learning Algorithm for Detection of Pneumonia on Chest Radiographs in Emergency Department Patients with Acute Febrile Respiratory Illness. J Clin Med, 9, 1981.
5 – Al aseri Z. (2009). Accuracy of chest radiograph interpretation by emergency physicians. Emerg Radiol, 16(2), 111-114.
6 – Hwang EJ, Park S, Jin K, et al. (2019). Development and Validation of a Deep Learning–Based Automated Detection Algorithm for Major Thoracic Diseases on Chest Radiographs. JAMA Netw Open. 2(3)
7 – Australasian College for Emergency Medicine. (2019). Quality framework for emergency departments Policy P28.
8 – Care Quality Comission. (2018). Radiology Review: A national review of radiology reporting. Newcastle upon Tyne
9 – Australian Institute of Health and Welfare 2014 Australia’s health 2014. Australia’s health series no. 14. Cat. no. AUS 178. Canberra: AIHW
10 – Kelly, C.J., Karthikesalingam, A., Suleyman, M. et al. (2019). Key challenges for delivering clinical impact with artificial intelligence. BMC Med, 17, 195.